Microsoft Introduces Aurora: The Dawn of a New Era in Weather Forecasting
Updated: June 11 2024 21:57In the aftermath of Storm Ciarán's devastating impact on northwestern Europe in November 2023, the urgent need for more accurate and reliable weather prediction models became abundantly clear. The storm's rapid intensification and record-breaking wind speeds exposed the limitations of even the most advanced AI weather-prediction models, as highlighted by a recent study by Charlton-Perez et al. (2024). As communities grappled with the destruction left in Ciarán's wake, a team of Microsoft researchers unveiled Aurora—a groundbreaking AI foundation model that promises to revolutionize our ability to anticipate and prepare for extreme weather events.
A Flexible 3D Foundation Model of the Atmosphere
Aurora is a 1.3 billion parameter foundation model designed for high-resolution forecasting of weather and atmospheric processes. Its effectiveness lies in its training on over a million hours of diverse weather and climate simulations, enabling it to develop a comprehensive understanding of atmospheric dynamics. By operating at a high spatial resolution of 0.1° (roughly 11 km at the equator), Aurora captures intricate details of atmospheric processes, providing more accurate operational forecasts than ever before—and at a fraction of the computational cost of traditional numerical weather-prediction systems.
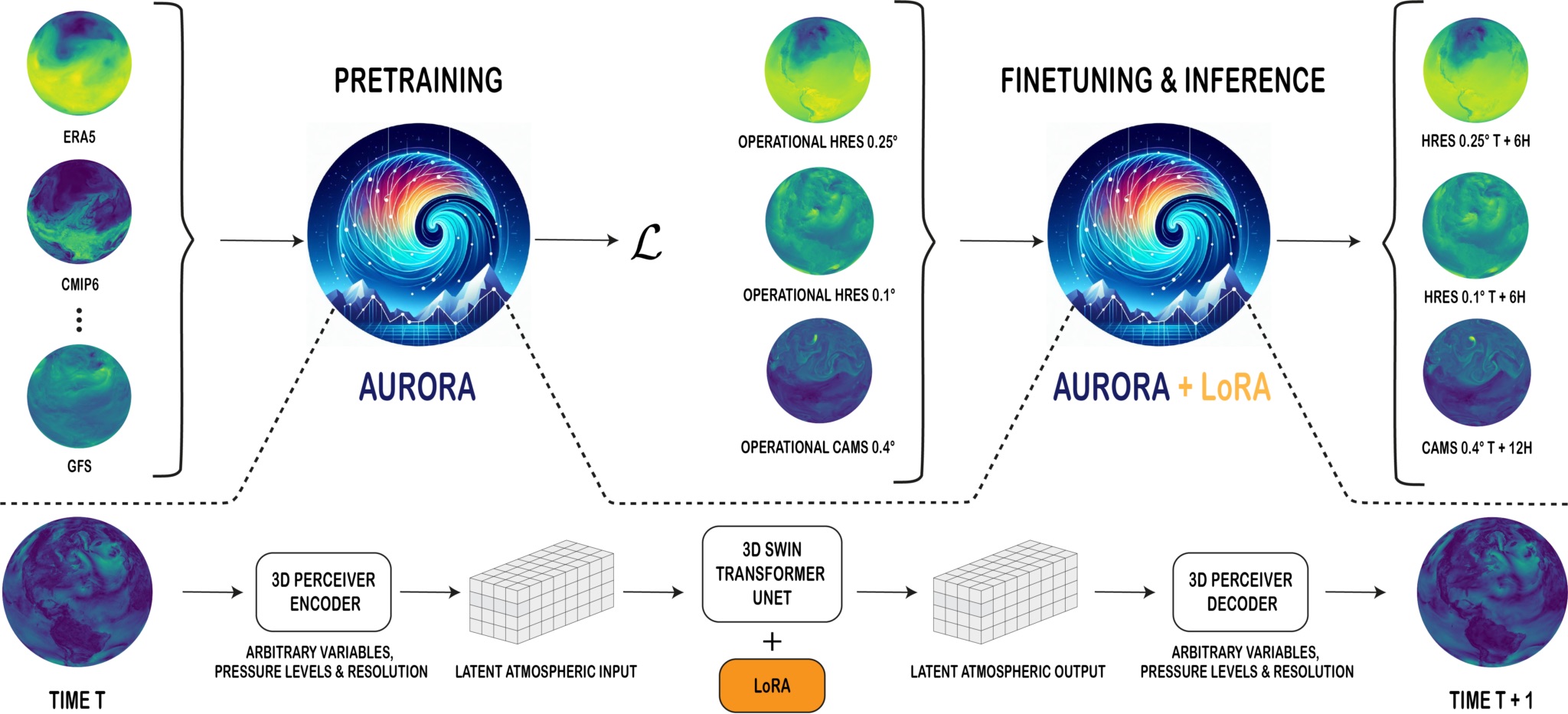
What sets Aurora apart is its versatility. The model can forecast a broad range of atmospheric variables, from temperature and wind speed to air-pollution levels and concentrations of greenhouse gases. Aurora's architecture, consisting of a flexible 3D Swin Transformer with Perceiver-based encoders and decoders, allows it to process and predict a range of atmospheric variables across space and pressure levels. By pretraining on a vast corpus of diverse data and fine-tuning on specific tasks, Aurora learns to capture intricate patterns and structures in the atmosphere, excelling even with limited training data when fine-tuned for a specific task.
Fast Prediction of Atmospheric Chemistry and Air Pollution
One prime example of Aurora's versatility is its ability to forecast air-pollution levels using data from the Copernicus Atmosphere Monitoring Service (CAMS)—a notoriously difficult task due to the complex interplay of atmospheric chemistry, weather patterns, and human activities, as well as the highly heterogeneous nature of CAMS data. By leveraging its flexible encoder-decoder architecture and attention mechanisms, Aurora effectively processes and learns from this challenging data, capturing the unique characteristics of air pollutants and their relationships with meteorological variables. As a result, Aurora produces accurate five-day global air-pollution forecasts at 0.4° spatial resolution, outperforming state-of-the-art atmospheric chemistry simulations on 74% of all targets.
Data Diversity and Model Scaling Improve Atmospheric Forecasting
A key finding of the Aurora study is that pretraining on diverse datasets significantly improves the model's performance compared to training on a single dataset. By incorporating data from climate simulations, reanalysis products, and operational forecasts, Aurora learns a more robust and generalizable representation of atmospheric dynamics. It is thanks to its scale and diverse pretraining data corpus that Aurora outperforms state-of-the-art numerical weather-prediction models and specialized deep-learning approaches across a wide range of tasks and resolutions.
Moreover, the study demonstrates that increasing model size leads to better performance for the same amount of computational resources. The researchers fit a power law that roughly translates into a 5% reduction in the training loss for every doubling of the model size, highlighting the importance of model scaling in improving atmospheric forecasting.
A Paradigm Shift in Earth System Modeling
The implications of Aurora extend far beyond atmospheric forecasting. By demonstrating the power of foundation models in the Earth sciences, this research paves the way for the development of comprehensive models that encompass the entire Earth system. The ability of foundation models to excel at downstream tasks with scarce data could democratize access to accurate weather and climate information in data-sparse regions, such as the developing world and polar regions. This could have far-reaching impacts on sectors like agriculture, transportation, energy harvesting, and disaster preparedness, enabling communities to better adapt to the challenges posed by climate change.
As the field of AI-based environmental prediction evolves, Aurora serves as a blueprint for future research and development. The study highlights the importance of diverse pretraining data, model scaling, and flexible architectures in building powerful foundation models for the Earth sciences. With continued advancements in computational resources and data availability, foundation models like Aurora could become the backbone of operational weather and climate prediction systems, providing timely, accurate, and actionable insights to decision-makers and the public worldwide.
Full Paper:
Aurora: A Foundation Model of the AtmosphereRecent Posts